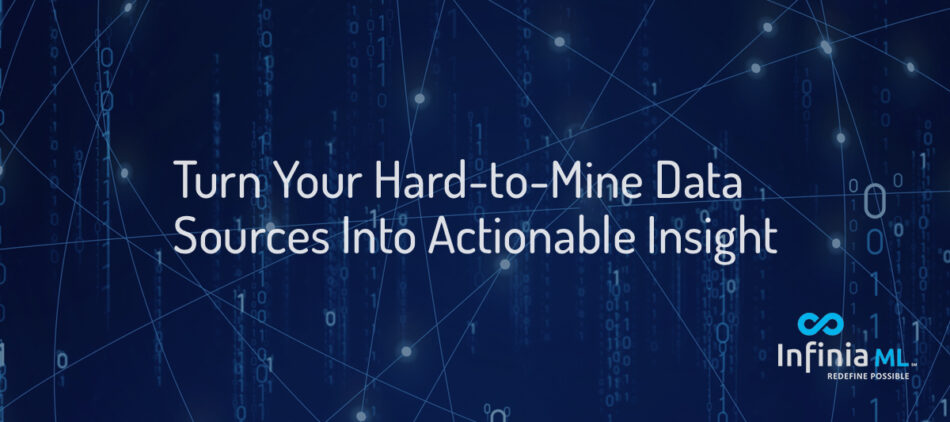
Turn Your Hard-to-Mine Data Sources Into Actionable Insight
Originally published on infinia on November 16th, 2021
A Conversation with Veritone’s Ryan Steelberg
Ryan Steelberg founded Veritone along with his brother in 2014. He serves as the company’s president and on its board of directors. Ryan has had an extensive career in tech with multiple exits. Before Veritone, Ryan was CEO of Brand Affinity technologies, head of Radio at Google after the acquisition of dMarc Broadcasting where he was president and co-founder, CEO of 2CAN Media, and President of AdForce, a company he co-founded while still an undergrad at UCLA.
- Ryan Steelberg, President of enterprise AI company Veritone, knows that it’s hard to get an AI project into production. He’s big on testing concepts with low-code tools as a first step before full-fledged production.
- ML is about more than just speed. By applying AI to data lakes consisting of documents and raw audio/visual files, machine learning can identify new insights and opportunities for growth not previously seen by the human eye. The precision of ML equips knowledge workers with details for targeted decision making that drive a company’s bottom line.
- To increase the odds of AI initiatives, leaders should identify intended outcomes, understand the available data, and look to low-code options for initial trial runs — ahead of production.
Body camera analysis and military satellite assessments might not be tasks you’d expect from a company that started in the media and entertainment industry. But that hasn’t stopped Ryan Steelberg. Veritone, where Ryan serves as president of an enterprise AI company changing perceptions of how AI can transform businesses.
Veritone partners with state and local law enforcement agencies to provide technology that analyzes raw body camera footage and quickly edits the film, protecting the anonymity of those in view. The technology allows agencies to promptly release video footage to the media while maintaining ethical standards.
It’s just one of many examples of how Veritone’s aiWARE Enterprise AI platform can support a diverse ecosystem of machine learning models, and help companies gain valuable insight from their data. Their work supports a range of industries, from media and entertainment to energy and government.
On a recent episode of Machine Meets World, Ryan and host James Kotecki discuss the new capabilities that AI can provide to businesses, how to ensure that data analytics projects make it to production and why combining documents and AV files into data lakes is a revelatory strategy.
AI’s Not Just About Speed
It’s common knowledge that AI can speed up processes, but the opportunities don’t stop there.
Machine learning can also sift through data and identify pieces of information, looking for signifiers that indicate an item needs further human-led analysis. It’s one way to address analyst fatigue, an increasingly pressing problem in many industries as data sets grow exponentially.
Additionally, while businesses come to Ryan with specific questions, he often finds that new insight is revealed as the ML is applied to the company’s data set. These insights open up further questions and opportunities that had not been identified by human analysis.
Ryan provides two examples of how AI’s helped his clients go deeper in their understanding of data and implementation of systems.
Identifying nuances in satellite surveillance data
- The U.S. Air Force has unfathomable quantities of raw satellite data. So much that the Air Force would need to hire 1.2 million people to analyze it all. That’s where AI comes in.
- Not only can AI technology cherry-pick the satellite data that needs to be reviewed by military professionals, but it can even scrutinize the data with detail not capable of the human eye.
- “The machines will pick up: hey, this [cargo ship’s] actually sitting a lot lower in the water than just two weeks ago,” says Ryan.
- It’s a feat that is dramatically transforming the U.S. Air Force’s capabilities and intelligence.
Zeroing in on the right (or wrong) host
In the entertainment industry, measurement is nothing new. Using TV viewership information to make critical programming decisions and determine ad placement is a decades-long practice.
But machine learning provides instant feedback and more profound insights than media executives could discern before the technology was available.
For example, “executives find out that this one host that they’ve just re-upped for another three-year contract has actually shown when they’re on screen, an increased breakage of people abandoning the show,” says Ryan.
The ability to pinpoint the influence specific individuals have on TV ratings empowers executives to make data-driven decisions on a micro level. Instead of just knowing what the ratings are, ML is helping them get to the bottom of what is driving viewers.
How exactly? The machine learning that drives Veritone’s capabilities is complex.
First, Veritone’s solution ingests all audio, video and other correlated structured data in real-time. This vast collection of data is then programmatically indexed. Structural metadata is added, along with a suite of cognitive services such as speech to text, logo and face detection. Veritone can then extract the company’s intelligent metadata, creating a core repository of primary-source data.
Once the data lake is developed, Veritone’s operating system allows easy access, through applications, APIs, or low-code tools, to insights that drive decision-making.
It’s an example of how artificial intelligence can make complicated tasks that involve tremendous volumes of information easily digestible and informative.
Documents Are the Cornerstone of Corporate Data
While Veritone deals primarily in audio and video data, Ryan emphasizes that documents still play an essential role in determining the ML output.
It’s a form of data that’s been around much longer than the AV offerings, making it an essential pillar in any AI initiative.
“Businesses have been building their business processes around documents for a very long time,” he explains. “How [business processes] function has been tightly correlated around documents, so you better know documentation, and you better know document flow if you’re going to start to think about including new datasets like unstructured audio, video or telephone calls.”
The more data, the more likely AI can generate new meaning and insight.
Often, leaders do not think there is a correlation between various sets of raw data, but when the company data is combined, and machine learning is applied, new insight is revealed that you wouldn’t have otherwise identified.
How a business process functions has been tightly correlated around documents, so you better know documentation, and you better know document flow if you’re going to start thinking about including new datasets like unstructured audio, video, or telephone calls.
How To Create a Successful AI Project — That Makes It to Production
It’s hardly an industry secret that most data strategy projects never make it to production. “AI is not magic,” Ryan explains when reflecting on why so many initiatives never see the light of day.
Implementing AI is a process that requires a lot more than funding. Companies investing in ML capabilities need to understand their goals while also committing to the work involved in initial iterations of the technology.
Ryan shares three pieces of advice for improving the odds that a business’s initiative will reach the final stages of development.
- “You better understand your data” he says. Leaders need to be able to speak to three questions:
- Is my business data-driven?
- What data is available to me?
- What information services do I currently use to understand data and drive decision-making?
- A business needs to know what it’s trying to achieve. “You have to come up with some problems that you want to solve,” Ryan says.
- Without intended outcomes, AI attempts will likely flounder. Set goals, such as increasing efficiency or bringing automation to the business that lowers headcount expenses by 10%. This will give developers concrete targets to hit.
- Before building a stack, use low-code tools to build workflow wireframes and test the theory.
- “Take advantage of those off-the-shelf tools to prove efficacy first, before you choose to take the next step,” suggests Ryan.
Veritone Voice at the Metaverse
It’s a buzzword these days with lots of hype, but Ryan believes in the promise of the metaverse.
He thinks AI will play a central role.
One area that has piqued his interest is the concept of digital personas, or avatars. To participate in metaverses, humans must create a digital version (or versions) of themselves.
At Veritone, they’ve developed Veritone Voice, a new AI capability that creates hyper-realistic synthetic voices. It’s an advancement that has many applications, including the ability for individuals to use their actual voice in digital spaces.
The technology is also able to take data from raw audio and transcribed text files and translate into 38 languages while preserving the original speaker’s voice. Which begs the question, which language should Machine Meets World try next?